COMPARISON OF PERFORMANCE AND COST
OF THREE SOLAR PHOTOVOLTAIC SYSTEMS
The purpose of this section is to compare the performance
of several solar photovoltaic (PV) systems made by different manufacturers
under actual operating conditions for residential applications. Another objective is to see how accurately
the performance of these systems is predicted by a publically available
software package developed by the National Renewable Energy Laboratory (NREL)
called “PVWatts 40 km Grid” (or Version 2).
This software computes the estimated AC power with corrections for the
PV module temperature's impact on PV efficiency, reflection losses, and
inverter efficiency as a function of load, in addition to the derate factors. The derate factors are discussed in more
detail below. This software package is the
most widely used computer model to size solar PV system to meet a specified
electrical load, and it is important that it be reasonably accurate.
These PV systems are all located within a few miles of
each other in a sunny, high mountain valley in or near Salida, Colorado
(south-central Colorado, zip code 81201).
All systems have been purchased and installed within the last two
years. The annual average solar
insolation on a flat plate collector tilted up from horizontal at an angle
equal to the latitude is about 5.78 kW/m2/day, according to the National
Renewable Energy Laboratory PV Watts Viewer (http://mapserve3.nrel.gov/PVWatts_Viewer/index.html). This may be compared with other locations in
the U.S. as shown for the same units (kW/m2/day) in Figure 1, which is also
from NREL (http://www.nrel.gov/gis/images/map_pv_national_lo-res.jpg),
and again this is for a collector tilted up at an angle equal to the
latitude. 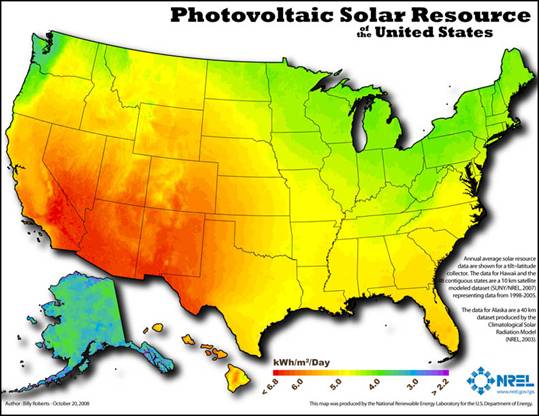
Figure 1.
Average Annual Solar Insolation on Flat Plate Tilted up at Angle Equal
to Latitude.
The three solar systems currently being monitored include
those shown in Table 1 below. These are
all standard production systems, and all were professionally installed. The first two systems, Sun Power and Sharp,
were installed in 2010, and the third one, REC, in 2011. Notice the large variation in PV module
efficiency, with the first one significantly higher than the other two. The cost per installed Watt before subsidies
has dropped from about $5.82 in 2010 to about $4.89 in 2011, with further
decreases in late 2011. The subsidized
cost varies depending on the utility supplier and the rebate program in place
at the time of purchase. The subsidized
cost is only 30% to 50% of the unsubsidized cost for these units, so the
subsidies are important in figuring payoff periods for solar PV. Table 1. Solar PV System Characteristics. Since the three systems all have different ratings, it
would be unreasonable to compare their total energy outputs directly. Rather, since the output power and energy
scale directly with the power rating at STC (standard test condition),
comparisons of performance are made by normalizing the output energy by the
rated power. This provides results in
energy/power, or kWh/kW.
From Table 1, it may be seen that the PV panels for
systems #1 and #2 are at the same orientation, being tilted up from horizontal
at 26.6˚ (for a 6:12 pitch roof) compared to a latitude of about 36.5˚, and
aimed 22˚ east of due south. As a first guess,
panels should be tilted up from horizontal at an angle approximately equal to
the latitude, and oriented due south. The
panels for system #3 are oriented further from the ideal orientation, being
tilted up from horizontal by 18.4˚ (for a 4:12 pitch roof), and aimed at 41˚
east of south.
The effect of aligning the panels in a direction other
than the optimum angle is not as strong as might be anticipated, as shown in
the figures below. In the first two
figures below, the effect of changing the tilt angle from horizontal (0˚) to
vertical (90˚) at the optimum azimuth angle of 169˚ (11˚ east of due south) is
shown, and in the next two figures, the effect of changing the azimuth angle
from due east (90˚) to due west (270˚) with the tilt fixed at the optimum angle
of 38.5˚ is shown. So Figure 2 shows the
PVWatts Version 2 predicted annual energy collected per kW of DC rating at
different tilt angles. Also noted in
Figure 2 is the energy corresponding to the common roof pitches used in
housing. Figure 3 shows the same
information but with the energy at various tilt angles expressed as a
percentage of the energy collected at the optimum tilt angle. It is interesting to note that all the common
roof pitches from 3:12 to 9:12 result in the collected energy of over 90% of
the optimum orientation at a fixed azimuthal angle.  Figure 2.
Effect of Tilt Angle (from Horizontal) on Annual PV Energy Generated per kW DC Power Rating for PV System, at
Azimuth Angle of 169 Deg. (11 E of S).
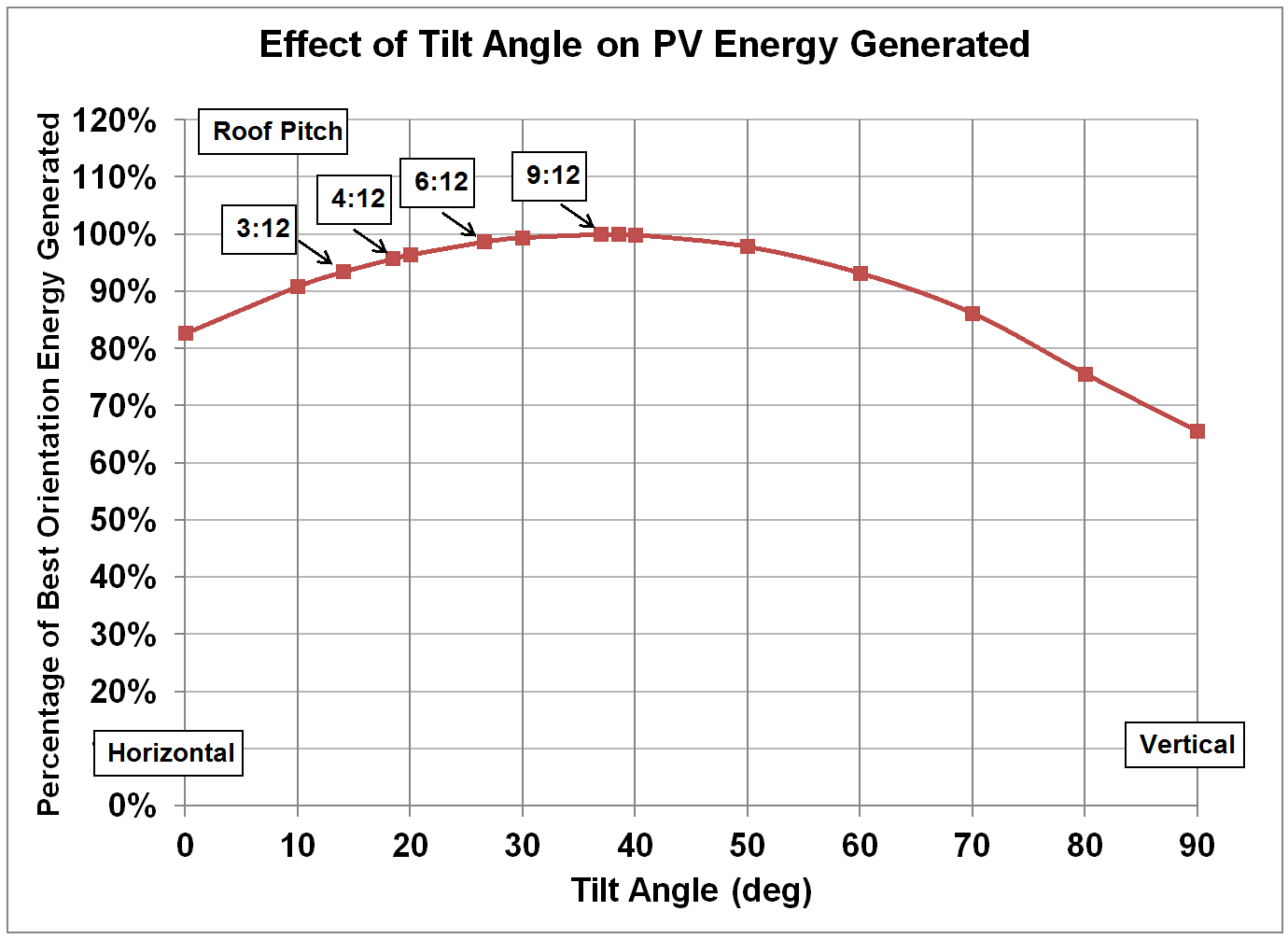
Figure 3.
Effect of Tilt Angle (from Horizontal) on Annual PV Energy Generated as
a Percentage of the Energy Generated at the Optimum Tilt Angle, at Azimuth
Angle of 169 Deg. (11 E of S).
Similarly, the effect of changing the azimuthal angle
(rotating the panels around a vertical axis) to point the panels from due east
to due west, while fixing the tilt at the optimum 38.5˚ is shown in Figures 4
and 5. Figure 4 shows the PVWatts
Version 2 predictions for annual energy collected per kW DC rating for various
azimuth angles. Interestingly, the
predicted energy does not peak at due south, but rather at 11˚ east of due
south. This result for optimum
orientation is due to two effects.
First, the air temperatures are lower in the morning, when the sun is in
the eastern sky, than in the afternoon, when the sun is in the western sky, especially
in this high mountain desert. Since PV
panel output decreases with increasing panel temperature, an eastern
orientation would be favored. Secondly,
the summer weather pattern in this Rocky Mountain Valley is for clear weather
in the morning, with clouds in the mid and late afternoon, so again, an eastern
orientation would be favored.
Figure 5 shows the same information as Figure 4, but expressed
as the annual energy generated at different azimuthal angles as a percentage of
the annual energy generated at the optimum angle of 169˚, all at a tilt angle
of 38.5˚. Note that the predicted energy
is at least 90% of the energy at the optimum angle for azimuthal angles ranging
from 120˚ (east-southeast) to 225˚ (southwest).
Thus, high PV output is predicted over a wide range of orientations
other than due south with tilt set to the latitude. Therefore, it is not necessary to wait until
a house is obtained with optimum roof orientation before installing a PV
system, or building a complicated rack mounting system to orient the panels differently
than the roof.
Figure 4.
Effect of Azimuthal Angle (due S = 180˚) on Annual PV Energy Generated
per kW DC Power Rating for PV System, at fixed Tilt of 38.5˚.
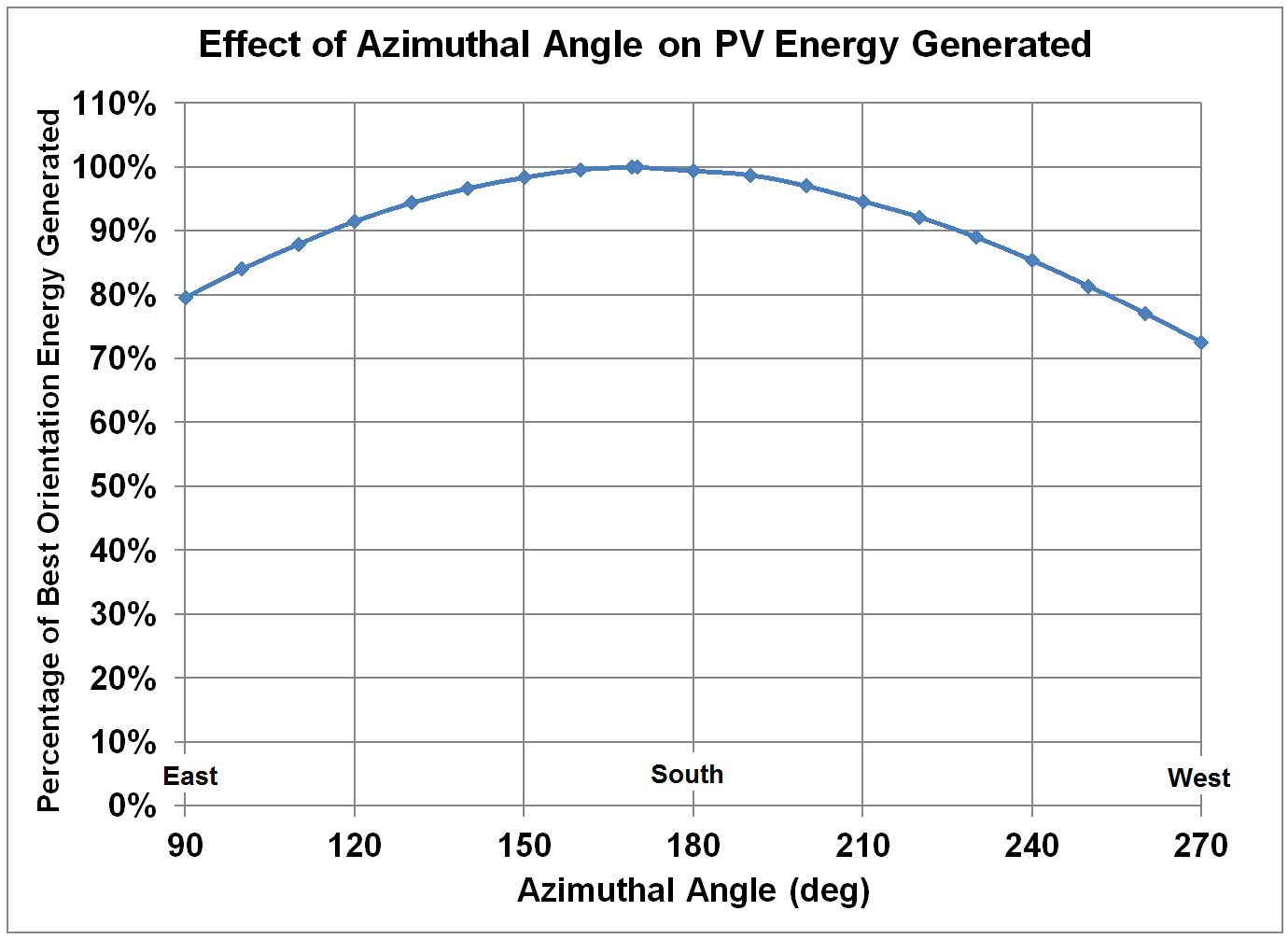
Figure 5. Effect
of Azimuthal Angle (due S = 180˚) on Annual PV Energy Generated as a Percentage
of Energy Generated at Optimum Azimuthal Angle, at fixed Tilt of 38.5˚.
It might be unreasonable to compare the normalized output
from each system directly, since systems #1 and #2 are at a more favorable
orientation than system #3. For that
reason, the output energy from each system is first normalized by the rated
power, and then compared with the computer model PVWatts Version 2 (40-km grid)
that accounts for the orientation of the panels as well as geographic location
(same for all three systems) to predict output energy for PV systems. By examining the measured energy output of
the PV systems and comparing it with the predicted output from PVWatts, the
different PV panel orientations can be approximately accounted for.
The other factor that must be included in predicting
performance of PV systems is aging of the solid-state solar cells in the PV
collectors. These three systems all use
silicon crystals, and the aging of monocrystalline and polycrystalline silicon
solar cells has been studied. A summary
of field degradation studies has been presented by Vazquez and Rey-Stolle
(2008), and they conclude that the yearly linear degradation must be less than
0.5% if the panels are to meet the 25-year warranty of at least 80% of the
rated power at that time. Based on their
literature survey and others, a linear degradation factor of 0.65% per year has
been selected for the results presented here.
Therefore, the PVWatts predicted energies and the projected field energy
measurements have been adjusted to reflect the 0.65% per year degradation
factor.
How can the aging factor be accounted for when using the
PVWatts computer model? The aging factor
is one of a number of factors that is included in the “derate” factors that are
used to adjust between the rated DC power at standard test conditions and the
expected power when operating in the field.
The standard aging factor is 1.0 (new array) as shown in Table 2, but
this can be adjusted downward as appropriate.
Also shown in Table 2 are the derate factors recommended by NREL for use
in PVWatts, as well as factors recommended by SunPower for their systems. For this work, the overall derate factor of
0.770 as recommended by NREL was used for the first year of operation, and then
this factor was reduced by 0.65% for each year beyond the first year.
Table 2.
Derate Factors for Use in PVWatts Computer Model, showing both the
Default Values Recommended by NREL and those Recommended by SunPower for their
Systems.
Loss Name
|
PVWatts
|
SunPower
|
Explanation of Loss
|
References
|
PV Module Nameplate DC Rating
|
0.950
|
0.980
|
Light-induced
degradation (LID), Deviation of actual module power from nameplate
|
1, 2
|
Inverter and Transformer
|
0.92
|
0.945
|
DC-to-AC energy
conversion loss in inverter and transformer
|
3
|
Mismatch
|
0.98
|
0.98
|
Power lost due to
non-optimal operating point of modules. Mismatch is minimized when module Isc
distribution is tight.
|
2
|
Diodes and Connections
|
0.995
|
1.000
|
Losses through
diodes external to the PV module junction box. Not applicable to most
SunPower systems. Losses through the diodes integral to the module are
included in the module flash test result.
|
|
DC Wiring
|
0.98
|
0.99
|
Loss in DC wiring
depends on system design. 0.99 loss is based on typical SunPower system
design.
|
4
|
AC Wiring
|
0.99
|
0.998
|
Loss in AC wiring
depends on system design. 0.998 loss is based on typical SunPower system
design.
|
4
|
Soiling
|
0.95
|
0.95
|
Soiling losses
are specific to region. SunPower uses 0.95 for arid regions and 0.99 for
rainy regions
|
5
|
System availability
|
0.980
|
0.980
|
No change from
PVWatts default
|
|
Shading
|
1.000
|
1.000
|
No change from
PVWatts default
|
|
Sun-tracking
|
1.000
|
1.000
|
No change from
PVWatts default
|
|
Aging
|
1.000
|
1.000
|
No change from
PVWatts default
|
|
Total
|
0.770
|
0.835
|
|
|
References for the derate table above: 1 – Photon Magazine article on LID 2 – Show table with up-to-date module flash test average
and distribution for modules most often sold into RLCC 3 – Include spec sheets for inverters most often used in
RLCC, or show table with CEC efficiency values for these inverters and
reference CEC website link. 4 – show representative electrical schematic of SunPower
system, including take-offs to show wire lengths and gauges. Show simple
calculation of ohmic losses in DC side based on average annual array current
from PVSim. Also show placement of inverter relative to breaker, and show
similar ohmic loss calculation for AC side. 5 – reference SunPower soiling papers from IEEE 2006 and
PVSEC 2007
Figure 6 shows the measured monthly
energy collected
divided by the rated DC power for PV systems #1 and #2 for
2011, Figure 7 shows the data for 2012, Figure 8 shows the
data for 2013, and Figure 9 shows the data for 2014. These systems are made by different
manufacturers and have very different efficiencies, but the measured monthly
energies normalized by the power ratings are
essentially identical within the scatter of the data. The energy collected by both systems exceeded
the predictions by PVWatts. Over the
first year of operation, system #1 exceeded the PVWatt’s prediction by
23.8%. Over the following years, the output continues to exceed PVWatt predictions.
Figure 10 shows the measured monthly
energy collected
divided by the rated DC power for PV system #3 for 2011, Figure 11
shows the data for 2012, Figure 12 shows the data for 2013, and Figure 13 shows the data for 2014. System #3 also appears to exceed the PVWatts
predictions. Because of the lower tilt angle for system #3
compared to the other two systems, the predicted power drops off more sharply
in the winter compared to the summer.
Figure 6.
Normalized Monthly Solar Energy Collected by Systems #1 and #2 compared
to Predictions by PVWatts with Default DC to AC Conversion Factor, but Adjusted
for Age for 2011.
Figure 7.
Normalized Monthly Solar Energy Collected by Systems #1 and #2 compared
to Predictions by PVWatts with Default DC to AC Conversion Factor, but Adjusted
for Age for 2012.
Figure 8.
Normalized Monthly Solar Energy Collected by Systems #1 and #2 compared
to Predictions by PVWatts with Default DC to AC Conversion Factor, but Adjusted
for Age for 2013.
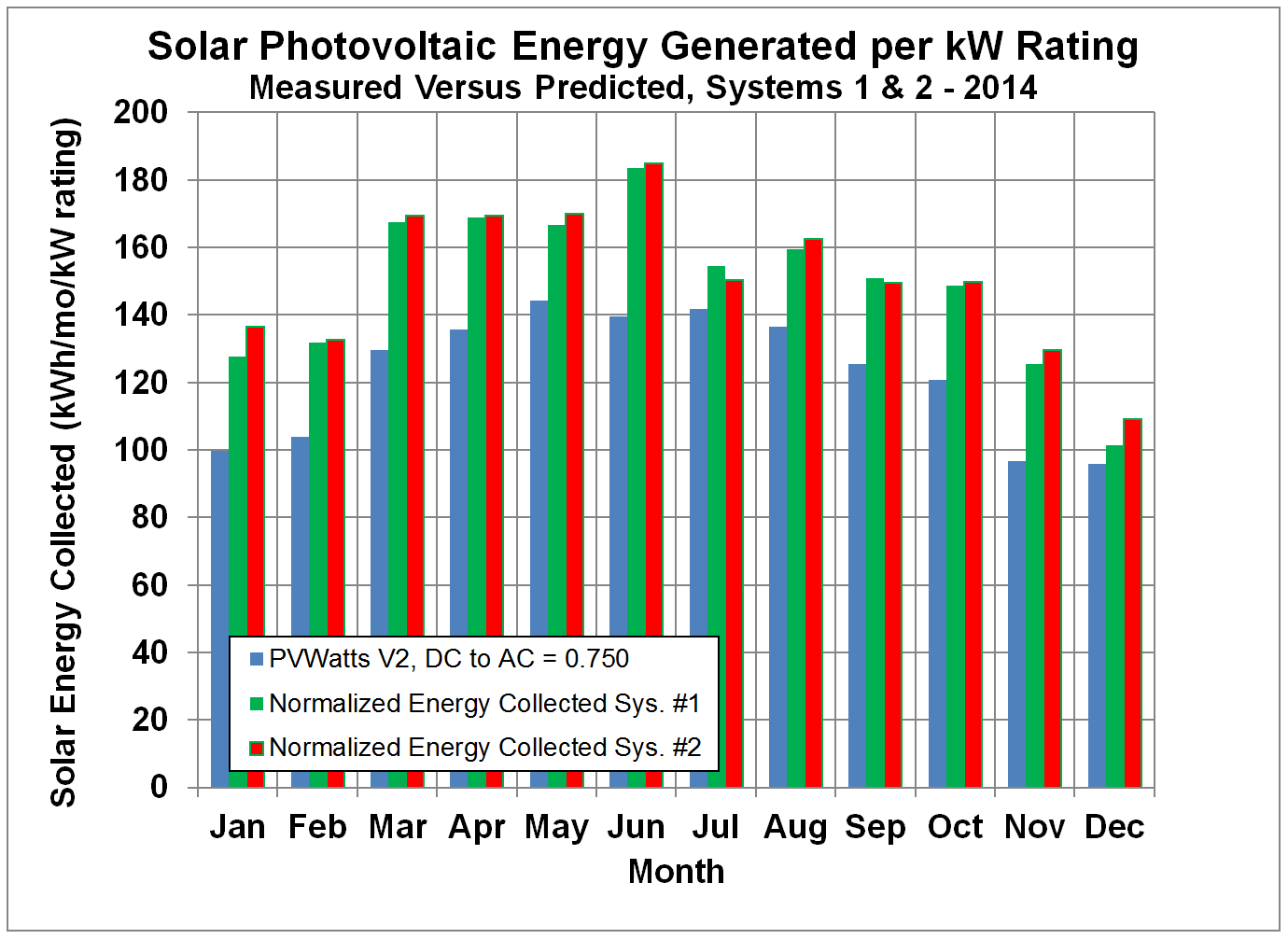
Figure 9.
Normalized Monthly Solar Energy Collected by Systems #1 and #2 compared
to Predictions by PVWatts with Default DC to AC Conversion Factor, but Adjusted
for Age for 2014.
Figure 10.
Normalized Monthly Solar Energy Collected by System #3 compared to
Predictions by PVWatts with Default DC to AC Conversion Factor for 2011.
Figure 11.
Normalized Monthly Solar Energy Collected by System #3 compared to
Predictions by PVWatts with Default DC to AC Conversion Factor for 2012.
Figure 12.
Normalized Monthly Solar Energy Collected by System #3 compared to
Predictions by PVWatts with Default DC to AC Conversion Factor for 2013.
Figure 13.
Normalized Monthly Solar Energy Collected by System #3 compared to
Predictions by PVWatts with Default DC to AC Conversion Factor for 2014.
Another way to compare the performance of different solar
PV systems is to take the measured energy outputs from the systems that are
shown in Figures 6 through 13, which have units of (kWh/mo)/kW rating and divide by
the days per month. This results in overall
units of hr/day, and can be interpreted as equivalent hours per day that the
system is producing AC power at the full DC rated power. Such a comparison is shown in Figures 14-17, which
show systems #1 and #2 to be performing about the same, just as in Figures 6 - 9,
with system #3 producing more energy in the summer months and less in the
winter, as expected from its lower tilt angle.
Figure 14.
Comparison of Normalized Output Energy from Three Solar PV Systems
expressed as Equivalent Hours of AC Power at the Rated DC Power Rating for 2011.
Figure 15.
Comparison of Normalized Output Energy from Three Solar PV Systems
expressed as Equivalent Hours of AC Power at the Rated DC Power Rating for 2012.
Figure 16.
Comparison of Normalized Output Energy from Three Solar PV Systems
expressed as Equivalent Hours of AC Power at the Rated DC Power Rating for 2013.
Figure 17.
Comparison of Normalized Output Energy from Three Solar PV Systems
expressed as Equivalent Hours of AC Power at the Rated DC Power Rating for 2014.
Comparisons
between PVWatts Predictions and Measured Energy
For the three systems tested, the actual measured collected
energy exceeded the PVWatts V.2 predictions by about 20% or more. It is not typical that actual energy
production is this much in excess of PVWatts’ predictions. Gostein et al. (2009) collected data from
over 480 residential and commercial installations of PV systems in Austin,
Texas, USA. They found that PVWatts
(V.1) predicted energy production was about 8% higher than the measured
energies of the PV systems.
Dean (2010) examined the discrepancies for model results
and concluded that the main area for discrepancies was not in model algorithms,
but rather in the input data for solar radiation. Because of variability in solar radiation
from day-to-day, month-to-month, and year-to-year, some method must be used to
select an average dataset or a typical dataset to use as input to the
simulation to compute electrical energy generated by the solar PV system. The radiation data used by NREL in PVWatts
comes from the Typical Meteorological Year data set, version 2, abbreviated as
TMY2. This dataset contains hourly
values of solar radiation, and is derived from 30 years (1961-1990) of
historical data for 230 locations across the USA from the National Solar
Radiation Database (NSRDB). Data for two
of the years were removed from the data set due to large volcanic eruptions
during those years. For each month, an
algorithm is used to select the “most typical” month of the thirty years in the
database. The algorithm minimizes the difference between the year in question
and the long-run average for each parameter. Dean points out some shortcomings of this
approach, and questions whether this “typical” dataset used to define TMY2
results in values that are “central,” or mean, or median.
Dean (2010) went back to the original NSRDB dataset that
includes the full 30 years worth of data, and processed those results using
quantitative risk analysis, which is a statistical approach to process the
large dataset. Further, he developed a
reduced form model using a neural network, as a part of this analysis. For the particular dataset that he chose in
Newark, New Jersey, his analysis showed that the PVWatts results using the TMY2
dataset resulted in a 6% higher energy production than his more complete
statistical analysis. Of course, to
accept Dean’s results requires faith in the neural network model, which is not
physically based, but it will be assumed that it was implemented
accurately. He suggests that for the
limited comparisons that he performed, that the TMY2 dataset was biased toward
higher solar radiation levels than average levels, and this was the source of
the high predictions by PVWatts (V.1).
Dean also points out that Peppers (2006) showed empirical evidence that
PVWatts (V.1) over-predicted field measurements by 10%.
Yates and Hibbert (2010) compare the performance of
several simulation codes with measured data for two locations in California. The PVWatts predictions for the first case
were 2.5% lower than the measured energy, while the PVWatts predictions for the
second case were 8.5% low relative to measured values. Enphase Energy in their advertising brochures
indicate that solar PV systems with their microinverters provide energy that
exceeds PVWatts predictions by an average of 8%.
Based on the above comparisons, the PVWatts predictions
are generally within ±10% of the measured values. Therefore, it seems odd that the three
systems tested in this location appear to produce more than 20% higher energies
than the PVWatts predictions.
It is likely the interpolation scheme used to estimate the solar
insolation for this local area does not properly account for the high
altitude of this location, and the higher solar insolation. It is likely that users in this immediate
area can expect performance significantly better than what is predicted by
PVWatts.
Application of
these Results to other Locations
The results shown here provide a guide to estimate PV
systems output energy based on the DC power rating. However, an additional parameter must be
considered to interpret these results, and that is the solar insolation (or radiation
or irradiation). The three systems shown
here all operate similarly in terms of collected energy per unit kW rating only
because they are exposed to the same solar insolation. To apply these results to other parts of the
country or the world, the results must be scaled based on the solar insolation
at the point of interest divided by the solar radiation these panels were
exposed to, which is a yearly average of about 5.78 kW/m2/day. See Figure 1 to estimate solar insolation for
other parts of the U.S., or use PVWatts V2 for a more precise value (http://mapserve3.nrel.gov/PVWatts_Viewer/index.html). For the U.S. the results presented here
should be roughly typical for the southwestern U.S., while other parts of the
country have lower solar insolation), and therefore, lower energy per DC kW
rating of the panels.
Economics for
Residential Solar PV Systems in Southern Colorado
The generation of electricity from solar PV panels has
generally not been cost competitive with electricity generated by large utility
plants in the past. Two things have
changed that have made the solar PV systems more cost competitive. First, significant subsidies from the federal
government and from local utilities have reduced the effective cost to
residential customers. Second, these
subsidies in the U.S., and sometimes even more generous subsidies outside the
U.S. have resulted in production increases, with the result that production
costs and retail prices have been reduced.
The federal government in the U.S. provides a 30% credit
for solar PV systems purchased for residential use. Utility subsidies are quite variable in both
time and place, but can be larger than the subsidies from the federal
government. Some states, mostly in the
northeastern U.S. also have solar renewable energy certificates (SREC’s) that
provide a market for the credits, providing an additional source of revenue for
owners of solar PV systems.
Of course, the solar industry is not alone is being a
subsidized energy source. There is the
Price-Anderson Nuclear Industries Indemnity Act that limits the nuclear
industry to pay only the first $12.6 billion in damages from a nuclear
accident. (The costs of the Japanese
tsunami-nuclear disaster have dwarfed this figure.) Also the research performed by the Atomic
Energy Commission, which was followed by the Energy Research and Development
Administration, which was followed by the Dept. of Energy is paid for
predominately or completely from federal taxes.
The oil industry has its three favorite deductions: (1) domestic
manufacturing deduction, (2) a deduction for treating royalties paid to foreign
governments as deductible taxes (sort of the opposite of the first deduction),
and (3) intangible costs deductions. This
list does not include the cost of foreign wars in oil rich nations, wars that
might be regarded as trying to secure future oil supplies. The war in Iraq has been estimated to have
cost about $1,000,000,000 U.S. dollars to date.
Thus, the argument that the economics of solar energy should be computed
on a non-subsidized basis would require that the costs for the competing energy
sources such as nuclear and oil also be adjusted for subsidies, probably an
impossible task. So the only realistic
comparison is for each energy source at the final cost to the consumer.
In spite of the subsidies for the U.S. oil industry, the
production of crude oil in the U.S. has declined dramatically since 1985, as
shown in Figure 18. M. King Hubbert (1956)
created and first used models to predict that United States oil production
would peak between 1965 and 1970. The
actual peak occurred 15 to 20 years later than his predictions, but just as he
predicted, the production has been steadily declining after this peak in
production. Similar models indicate that
peak oil production world-wide will occur around the present time (2010). This decline in crude oil production emphasizes
the need to find renewable energy sources, and provides a motivation for
studying solar energies such as PV systems for electrical generation.
The costs to the homeowners for the PV systems are known,
but maintenance costs are unknown. From
examining the literature and discussions with a solar PV installer, it is
assumed that the inverters for the PV systems will last 12 to 15 years, so that
the inverters will require replacement once over 25 years. The cost for a SunPower 3000m inverter is
about $2312, and a labor cost of $500 has been estimated for replacement. Additional maintenance over 25 years has been
estimated at $500. All of these costs
are listed in Table 3, and they can be summed to estimate a total cost for 25
years of operation, with the further assumption that the system is obsolete
after 25 years. If the energy output for
these systems can be estimated for the same period, then the cost per unit
electrical energy can be computed and compared with commercial rates.
Figure 18.
Production of Oil in the United States.
The energy output from system #1 has been monitored for
over a year. The energy output for
system #2 has been monitored for a shorter time, but it seems to produce the
same energy per kW rating as system #1 as shown in Figures 6 - 9. The output from system #3 relative to the
PVWatt’s model predictions is similar to systems
#1 and #2, as shown in Figures 10 - 13.
Therefore, the annual performance of system #1 can be used to estimate
the output of system #2 by just scaling based on the rated system power. The performance of system #3 can be estimated
as the annual output from system #1 scaled by the predicted annual energy from
PVWatts for the two systems including the effect of the different power ratings. These estimated annual energy outputs have
been extrapolated to 25 years worth of performance, with each year linearly
decreased from the previous year by 0.65%, the annual degradation factor
discussed above, with results presented in Table 3.
Since both the PV system costs and output energies have
been estimated as shown in Table 3, the cost per kWh can be computed. Those results are shown in Table 3 as “Cost
per solar kWh over 25 years.” Those
results are compared with the electricity costs when purchased from the
appropriate utilities, and these results are shown in Table 3. The cost from utilities is different for
system #3 than for #1 and #2 since a different utility serves the respective
areas. In this part of southern
Colorado, a homeowner can only choose the one utility that offers service in
the area.
Table 3. Initial Costs, Estimated Maintenance Costs,
Estimated Performance, and Energy Costs for the Three Solar PV systems.
As can be seen in Table 3, the cost for the solar PV
generated electricity amortized over 25 years is less than the current cost of
electricity from the utilities. However,
most people do not live in their homes for 25 years. Thus, to recoup the initial investment, the
value of the home would have to reflect the value of the solar PV system. A report by Hoen, et al. (2001) reports that
homes in California with solar PV systems sold at a premium of about $5.50/watt
(approximately the unsubsidized cost of a PV system) compared to comparable
homes with PV systems, or roughly the cost of a new PV system. Most of the surveyed homes had relatively new
PV systems with a typical size of 3.1 kW, and the price premium tended to
decrease for older PV systems, as would make sense. Since the PV market is larger in California
than in any other states, caution must be used in extrapolating these results
to other states. However, it is
reasonable to expect that as solar PV systems become more widespread, this same
increase in resale home value with PV systems might be expected.
Another way to look at cost analysis of PV systems is to
compute the payback period, that is, how many years will it take to pay back
the initial investment. If a simple
payback calculation is performed, it ignores the potential increase in energy
prices, as well as the cost of money for the initial investment. (These assumptions are explored below.) In this analysis, the projected decrease in
PV system output of 0.65% per year is included.
The simple payback for system #1 is 9 years, for system #2 is 12 years,
and for system #3 is 11 years. After
this payback time, the systems provide “free” electricity for the balance of
the 25-year lifetime, except that a first inverter replacement would be a
likely additional cost at 12 to 15 years after installation. The panels are guaranteed to still produce
80% of rated power at 25 years, so the actual lifetime is expected to be longer
than 25 years, but a second inverter replacement would need factored in for longer
lifetime projections.
The simple cost analysis shown in Table 3 does not
include the likely inflation rate for electricity costs, nor the cost of money
used to invest in the PV systems. Some
comments can be made concerning these factors.
Estimating inflation rates into the future is difficult given the
background of the very high inflation rates during World War I and II and of
the 1970’s contrasted with the low inflation rates of the 1930’s, the 1950’s,
and more recent years. The average
retail price of electricity to residential customers over the period 1997 to
2009 is shown in Figure 19, and the average inflation rate as computed using a
least-squares error fitting procedure is computed to be 3.07%. Much of the new generating capacity uses
natural gas as the fuel of choice, and the average retail price of natural gas
to residential customers is shown in Figure 20 for the period 1989 through
2010. Using a least-squares error
fitting procedure, the natural gas inflation rate over this period was 4.69%. A cap-and-trade policy to reflect the cost of
build-up of greenhouse gases in the atmosphere might cause the energy inflation
rate to increase at a faster rate than the past historical rate.
How do these inflation rates for energy compare to
overall inflation rates for all commodities?
The consumer price index from 1913 through 2010 is shown in Figure 21. It is clear that over that period,
inflation rates have varied dramatically, with higher inflation rates during
major wars and the energy crisis of the 1970’s, and lower rates during
recessions and depressions. The average
inflation rate over the extended period from 1913 through 2010 was computed to
be 3.58% using a normalized least-squares error fitting. However, the inflation rate over the more
recent periods corresponding to the data shown for electricity in Figure 19 and
natural gas shown in Figure 20 are lower.
For the period 1997 through 2009, the CPI inflation rate was 2.68%
compared to the inflation rate for electricity of 3.08%. For the period 1989 through 2010 the CPI
inflation rate was 2.64% compared to the inflation rate for natural gas of
4.69%.
The conclusion is that energy prices appear to have an
inflation rate similar to, but higher than, (much higher for natural gas) the
overall inflation rate as measured by the CPI.
It is possible that with “peak oil” already having occurred, and with
high growth economies in Asia and South America, that future energy prices will
escalate at a faster rate that what has occurred over the last decade or two,
but this is only speculation at this point.
Currently long term investments in bank accounts draw
about 1% or less, while long-term mortgages are at about 3.5%. Therefore, the cost of money is similar to
the overall inflation rate, and also similar to, or lower than, the inflation
rate for energy. Thus, the simple
analysis above using fixed 2011 U.S. dollars is a reasonable first step for
estimating return of solar PV systems, although the actual return might be
better than shown if the cost of energy continues to inflate at rates higher
than the overall cost of living.
It should be noted that solar PV systems my now be leased
in many parts of the U.S. and perhaps in the rest of the world. In some cases, the lease agreements require
no up-front cost by the homeowner, but only a monthly payment. Analysis of these lease agreements is outside
the scope of this report, but might be an attractive option for homeowners
wanting to avoid the large up-front investment.
The economic analysis presented here is influenced by the
following factors: initial system cost, solar insolation (5.78 kW/m2/day in
this area), utility rates, and rebate amounts by governments and utilities. So these factors should be taken into account
in using these results to apply to other parts of the world.
Figure 19.
Average Retail Price of Electricity to Residential Customers in the
United States over the Period 1997 – 2009.
Figure 20.
Average Cost of Natural Gas to Residential Customers in Colorado over
the period 1989 – 2010.
Figure 21.
The Consumer Price Index over the Period 1913 thorough 2010, and
Inflation Rates Calculated for Various Time Periods.
References:
Dean, S.R. (2010). “Quantifying the Variability of Solar PV
Production Forecasts,” SOLAR 2010 Conference Proceedings.
Gostein, M., Hershey,
R., Dunn, L., Stueve, B. (2009). “Performance
Analysis of Photovoltaic Installations in a Solar America City,” Photovoltaic Specialists Conference (PVSC), 2009 34th IEEE.
Hoen, B., Wiser, R., Cappers,
P., and Thayer, M. (2011). “An Analysis
of the Effects of Residential Photovoltaic Energy Systems on Home Sales Prices
in California,“ Lawrence Berkeley National Laboratory report LBNL-4476E. Download
from http://eetd.lbl.gov/ea/emp/reports/lbnl-4476e.pdf.
Hubbert, M. K. (1956). "Nuclear Energy and
the Fossil Fuels 'Drilling and Production Practice'". Spring Meeting of the Southern
District. Division of Production. American Petroleum Institute. San Antonio, Texas: Shell
Development Company.
pp. 22–27. http://www.hubbertpeak.com/hubbert/1956/1956.pdf.
Marion, B., Anderberg, M.,
George, R., Gray-Hann, P., and Heimiller, D. (2001). “PVWATTS Version 2 – Enhanced Spatial Resolution for Calculating
Grid-Connected PV Performance,” NREL Report NREL/CP-560-30941, presented at the
NPCV Program Review Meeting.
Pepper, J. (2006). Comments of Clean Power Markets, Inc. on CPUC
Energy Division Staff Draft Proposal Phase I for the California Solar
Initiative Design and Administration 2007-2016, Filed under Rulemaking
06-03-004, CPUC, May 16, 2006
Vazquez, M., and Rey-Stolle, I. (2008). “Photovoltaic Module Reliability Model Based
on Field Degradation Studies,” Prog. Photovolt: Res. Appl. Vol. 16: 419-433.
Yates, T., and Hibbert, B. (2010). “Production Modeling for Grid-Tied PV
Systems,” Solar Pro, pp. 30-56,
April/May, 2010.
|